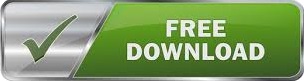
The most predominant oscillatory activity in the EEG/MEG is at the alpha rhythm (8–12 Hz), which has been observed primarily in the sensory systems (vision, motor, auditory). Here, using various simulations modelling the common task induced changes in the EEG reported in the literature, we demonstrate that apparent changes in Inter-Trial Phase-Locking of oscillatory activity can occur independent of any actual change in the phase of the ongoing activity.ĮEG and MEG signal contains oscillatory (i.e rhythmic) activity in various frequency bands. One measure, Inter-Trial Phase-Locking (ITPC), or the uniformity of phase angles across trials, is particularly vulnerable to these factors. A possible source for the discrepancy could be that the estimation of the phase of ongoing oscillations as well as its uniformity across trials could be affected by task induced changes in the power of oscillations or concurrent evoked responses. However, studies examining whether the adjustment of the phase angle of oscillations is under top-down attentional control have thus far yielded conflicting results.
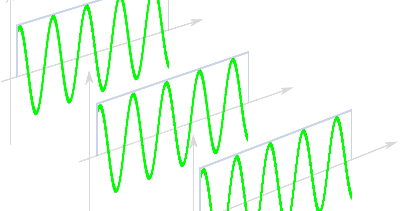
In this case, the obtained eigenvalue spectrum exhibits a decrease in the last eigenvalue and a compensatory increase in the lower eigenvalues compared to case (b), which consequently yields a meanλ 1:60% value between those in cases (a) and (b).Many studies have now consistently reported that the phase angle of ongoing oscillatory activity (measured using EEG/MEG), at time of stimulus presentation influences detection when stimuli are near-threshold. (c) A set of IMFs extracted from different channels within a 1-s ictal period leads to a R N×N matrix with values between case (a) and (b), showing a partial synchronization among the oscillators. In this case, the eigenvalue decomposition of the R N×N matrix yields five for the last eigenvalue and zero for the rest of the eigenvalues.
#MEAN PHASE COHERENCE FULL#
(b) Five-times copied version of an IMF leads to a R N×N matrix, in which all of its values are equal to one, showing a full phase-synchronization among the oscillators. In this case, all the eigenvalues resulted from the eigenvalue decomposition of the R N×N are close to one. Due to the nearly orthogonal nature of the IMFs extracted from one channel, all the R N×N values are near to zero, showing no phase-synchronization among the oscillators. (a) Five decomposed IMFs from a 1-s, bipolar-derivate ECoG signal using the NA-MEMD process, along with their corresponding mean-phase coherence matrix, eigenvalue spectrum, and the average value of the first 60% lower-index eigenvalues, meanλ 1:60%. All of the values in the mean-phase coherence matrix, R N×N, were normalized and color-coded between zero (black), to one (white).
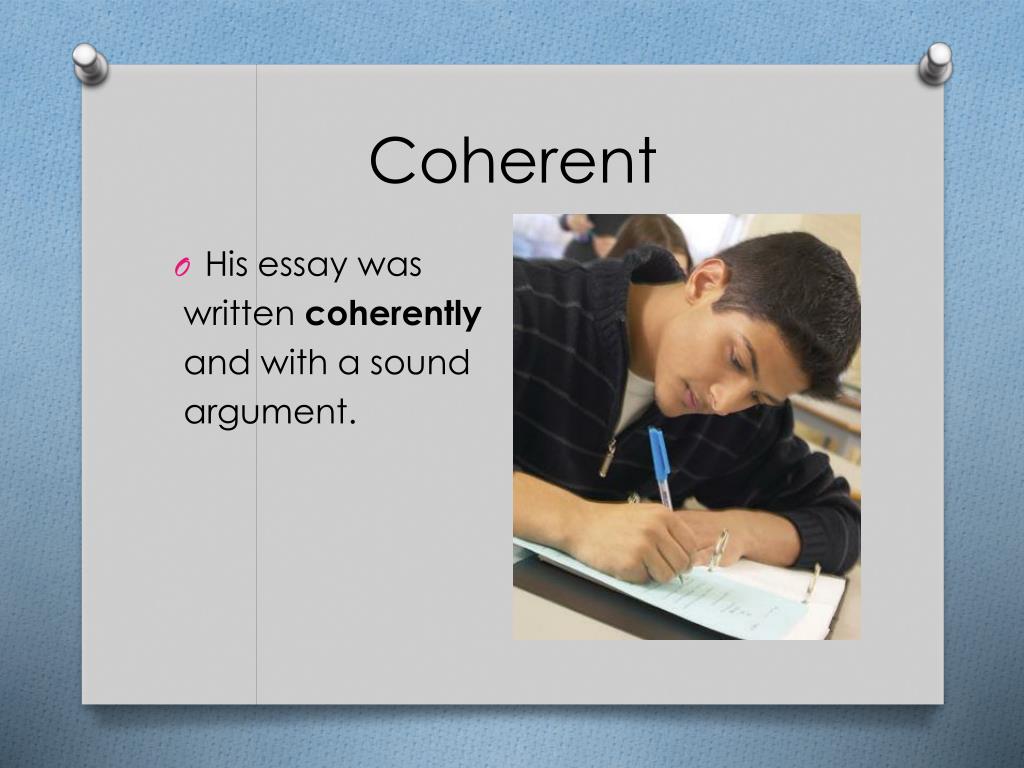
This result suggests that hyper-synchronization of the epileptic network may be an essential self-regulatory mechanism by which the brain terminates seizures.Įvaluating phase-synchrony levels among neuronal oscillators using eigenvalue decomposition of the mean-phase coherence matrix. However, the network phase-synchrony started to increase toward seizure end and achieved its maximum level at seizure offset for both types of epilepsy. Drug-refractory patients with frontal and temporal lobe epilepsy demonstrated a reduction in phase-synchrony around seizure onset. The extracted neuronal oscillators were grouped with respect to their frequency range into wideband (1-600 Hz), ripple (80-250 Hz), and fast-ripple (250-600 Hz) bands in order to investigate the dynamics of ECoG activity in these frequency ranges as seizures evolve.
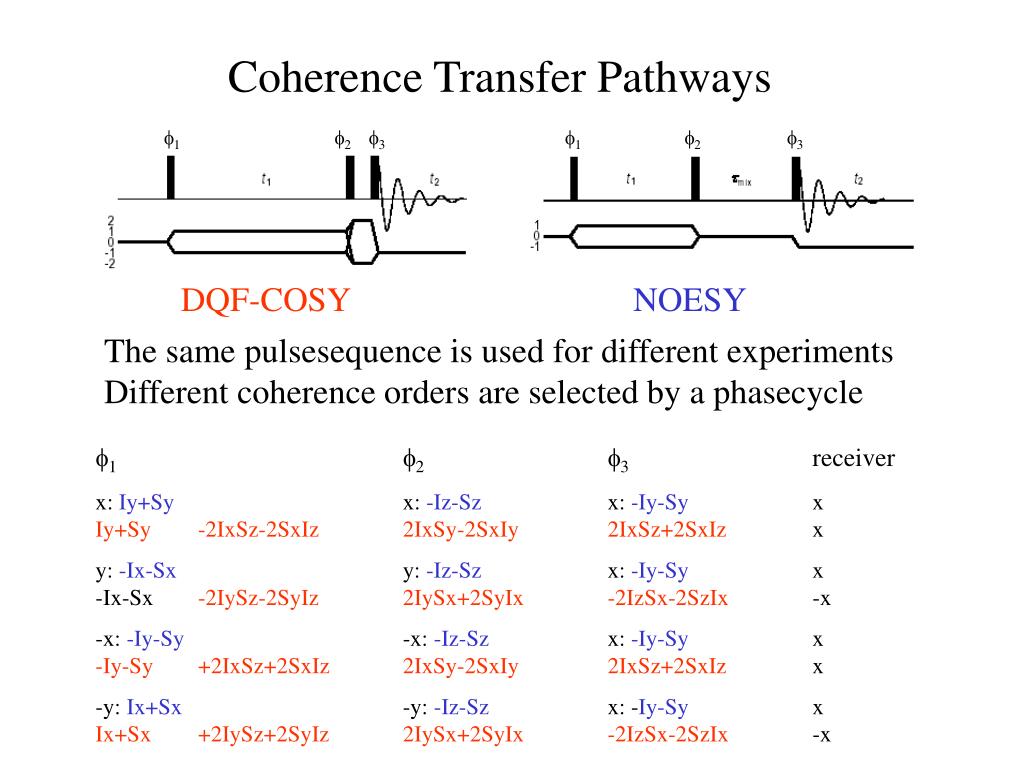
The phase-synchrony dynamics were then assessed using eigenvalue decomposition. Next, the instantaneous phases of the oscillatory functions were extracted using the Hilbert transform in order to be utilized in the mean-phase coherence analysis. A set of finite neuronal oscillators was adaptively extracted from a multi-channel electrocorticographic (ECoG) dataset utilizing noise-assisted multivariate empirical mode de-composition (NA-MEMD). In this paper, a non-linear analytical methodology is proposed to quantitatively evaluate the phase-synchrony dynamics in epilepsy patients. Spatiotemporal evolution of synchrony dynamics among neuronal populations plays an important role in decoding complicated brain function in normal cognitive processing as well as during pathological conditions such as epileptic seizures.
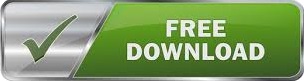